Despite improvements to the current available treatments for breast cancer, determining an accurate prognosis to guide treatment decisions remains a challenge. One approach that could help improve patient care is predictive oncology. This approach is a shift from traditional cancer treatments, instead taking patient-specific data into account in a more precise way so that oncologists can better understand how tumors will respond to treatments.
When combined with computational modeling (Figure 1), predictive oncology can be used to anticipate treatment outcomes for patients using algorithms and machine learning techniques. For instance, mathematical equations that describe the biological and physical mechanisms behind cancer growth, as well as treatment responses, can be used to create deterministic models of tumor progression. One company that is using modeling in this way is initiatives for Bio-Materials Behavior (iBMB Srls), a spin-off of the University of Basilicata. iBMB Srls used the equation-based modeling functionality of the COMSOL Multiphysics® software to create CancerMate, a simulation app that is based on a mathematical model that represents tumor behavior.
The intended focus of the CancerMate app is to simulate the proliferation of cancer cells in patients with nonmetastatic triple-negative breast cancer and to virtually quantify lesion volumes before they undergo neoadjuvant LYNPARZA® (olaparib) chemotherapy to reduce tumor size. With the results from the app, oncologists can better monitor solid tumor progression and adjust therapeutic strategies accordingly.
"The current treatments on the market lack personalization and precision," said Gianpaolo Ruocco, CEO of iBMB Srls. "CancerMate allows doctors to run virtual scenarios, reducing the burden on the patient and the cost of the treatment."
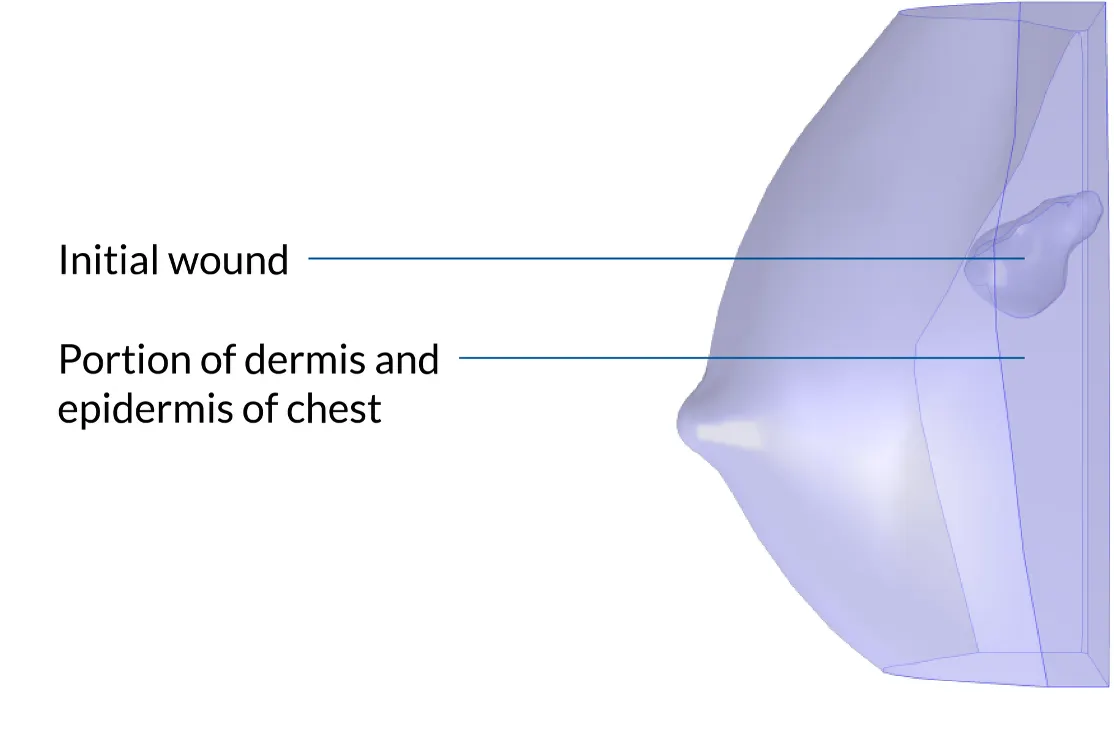
Determining Tumor Volume via Virtual Biomarkers
The CancerMate app was validated against data from a clinical experiment conducted with patients treated with LYNPARZA® for nonmetastatic triple-negative breast cancer. The clinical experiment helped the team identify key breast carcinoma biomarkers, including tumor-infiltrating lymphocytes (TILs), which describe immune response, and protein Ki67, which describes tumor aggressiveness. During the study, these biomarkers were closely monitored over time.
The data from the experiment was used to test a mathematical model built to predict the metabolic response of breast cancer, virtualize tumor progression, and predict tumor dynamics in response to therapy for individual patients.
CancerMate: A Leap Toward Personalized Oncology
The CancerMate app uses this mathematical model to integrate patient data that includes baseline measurements such as metabolic tumor volume, TILs, and Ki67. In the CancerMate app, virtual biomarkers are integrated into models as digital or computational indicators that represent biological processes or disease characteristics, intended to supplement or predict traditional clinical biomarkers. The virtual biomarkers for personalized malignancy and personalized pharmacodynamic efficiency inform the model's predictions, and then the app will apply these biomarkers to a set of equations that describe tumor growth and response to treatment over time.
The user interface of the app includes input fields for patient-specific data, such as the total observation period, patient mass, body surface area, baseline Ki67 and TIL values, dosage, and creatinine levels (an indicator of how the body disposes the drug via bodily functions).
After computing, the numerical results for the predicted clinical lesion values are displayed, and a graphic shows the progress of the predicted cancer lesion volume and the integrated drug concentration.
Ruocco and his team created the app with the Application Builder in COMSOL Multiphysics and then, using COMSOL Compiler™, converted it to a standalone format that allows the team to easily distribute the app to clinicians, enabling them to perform virtual scenarios and receive detailed information about cancer progression at their desktop (Figure 2).
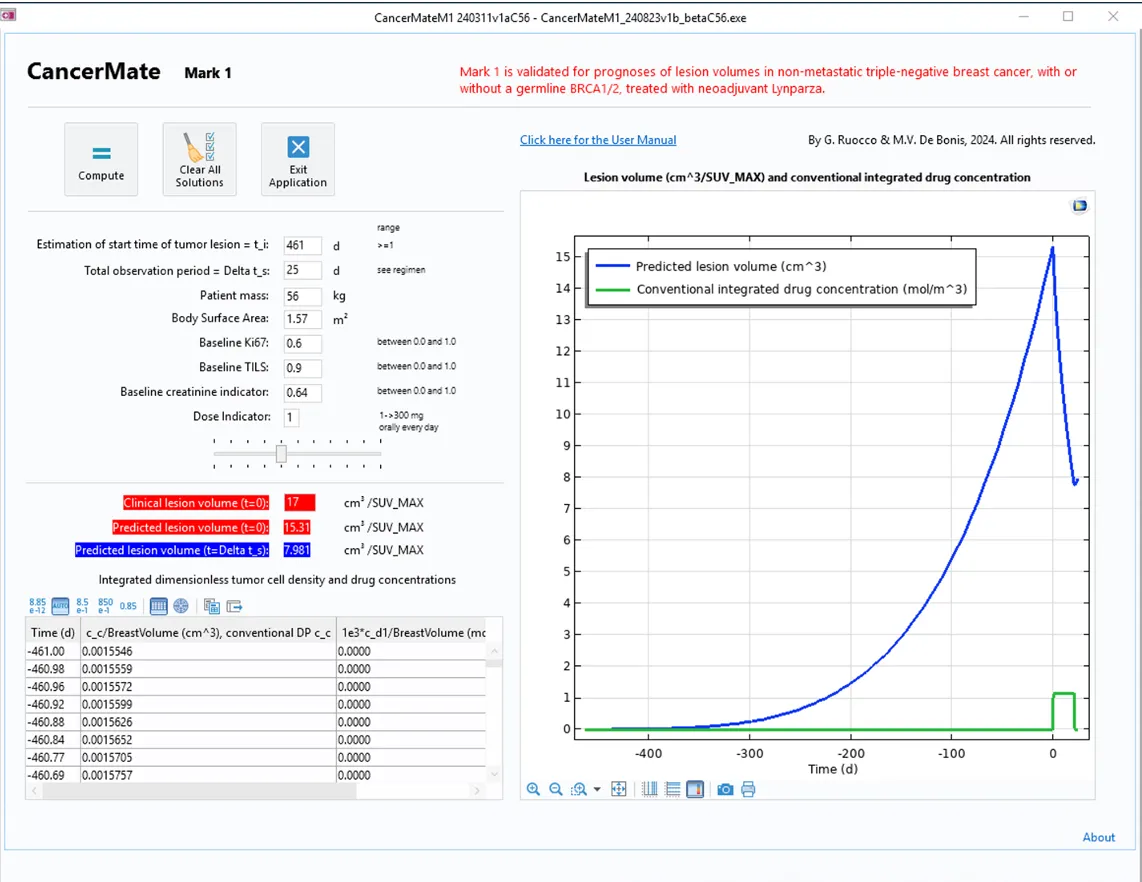
CancerMate's user-friendliness and simulation capabilities make it valuable in personalized cancer treatment for oncologists and pharmaceutical researchers. The current version is ready for implementation in a clinical setting for triple-negative breast cancer treated with LYNPARZA®, and Ruocco said that the app's underlying technology could be trained to cover more breast cancer subtype–therapy pairings and extended to other types of cancer and drug products.
As predictive oncology evolves, tools like CancerMate can shape personalized medicine by helping clinicians assess and monitor tumor lesion volumes. "Patients are often treated longer than necessary, but this can be modified with in silico tools," explained Ruocco.
LYNPARZA is a registered trademark of AstraZeneca AB.